A data scientist’s view of AI
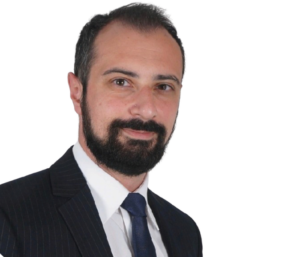
At SaaScada we’re always talking about the importance of data and the many roles it plays within a financial institution, from protecting the business and its customers, through to driving innovation. But this time we thought we would ask a data scientist for his view on the topic.
Our guest writer, Angelos Markos, has spent over a decade delving into data within the financial services industry and is as passionate about the subject as we are. Given artificial intelligence is such a hot topic right now, he has shared the data scientist’s view of the potential role artificial intelligence and machine learning can play in financial services.
Transaction Outlier Detection
The core of financial security revolves around identifying and mitigating fraudulent activities. AI and ML stand at the forefront, offering sophisticated algorithms capable of detecting outliers in transaction data. Unlike traditional methods, AI-driven systems learn from historical data, adapting to new fraudulent patterns as they evolve. For instance, a bank uses AI models to monitor its customer transactions. One customer, typically purchasing locally, suddenly makes a high-value transaction in a foreign country. The AI system flags this as an outlier based on the customer’s historical data, initiating a verification process to prevent potential fraud.
Real-time Anomaly Detection
Anomaly detection in real-time is a game-changer for the financial industry. This technology not only identifies potential fraud but does so as transactions occur, offering a proactive approach to security. By integrating SaaScada’s real-time data, AI models can monitor for anomalies with unprecedented accuracy, safeguarding against sophisticated cyber threats. Consider a scenario where an online banking platform experiences a sudden spike in transactions at an unusual hour. Real-time anomaly detection systems, powered by AI, can immediately identify this pattern as suspicious, potentially halting a cyber-attack in progress.
Predictive modelling
The same outlier detection that can flag threats, can also be used to drive increased customer satisfaction. For example, where branches have periodic increases in demand due to proximity to large events or in areas with seasonal tourism real-time data can ensure that the frequency of cash orders can ensure ATMs don’t run low on cash. Once the system has identified this trend, it is possible to combine external data with inhouse data to identify the trigger and ensure these anomalies/trends are catered for in the future. time, This proactive approach transformed the data scientist’s role from a passive observer to an active participant in customer satisfaction.
Tailored Financial Products through AI
The personalization of financial services is a significant trend, with AI at its core. By analysing transaction data, AI algorithms can offer customized product recommendations via basket analysis that resonate with individual consumer behaviours and preferences enhancing the engagement of customers with the financial service. Imagine a personal finance app that analyses a user’s spending habits to recommend a savings plan. If the user frequently dines out, the app, using insights from transaction data, could suggest a cash-back credit card for dining. This personalization is made possible by analysing transaction data to understand individual preferences.
Auto-mapping in Data Organization
The organization of vast amounts of unstructured data into a coherent structure is a daunting task for financial institutions. Here, AI and ML technologies offer a solution through auto-mapping, which automates the categorization of data. This process not only enhances operational efficiency but also improves the accuracy of financial reporting and analysis. For example an data management tool automatically categorizes transactions into ‘groceries,’ ‘utilities,’ and ‘entertainment’ using AI. This simplifies users’ budgeting efforts, allowing for a clearer understanding of spending habits without manual data entry, showcasing the practical benefits of auto-mapping.
Utilising Generative AI
Generative AI represents the cutting edge of AI research and its application in financial services is promising. This technology can simulate financial scenarios, generate personalized advice, and model the behaviour of customers to create target groups for the development of new products. It can truly explore the art of the possible, by utilising data without the personal biases or experiences of the individual, to posit scenarios or products that don’t yet exist but are based on emerging trends and behaviours identified in the data. This would enable a data scientist to use this technology to work with the product development team to simulate and develop innovative digital banking products tailored to younger, tech-savvy demographics, foreseeing and shaping future banking habits.
In the case of risk management, a bank can employ Generative AI to simulate various economic downturn scenarios. These simulations help in stress-testing the bank’s portfolio against potential future crises, preparing for a range of outcomes. Another application is virtual financial advisors, powered by Generative AI, taking the existing algorithm-driven model portfolios to the next level by offering personalized investment advice by simulating market conditions and predicting future trends based on current data.
Making AI work for you – data is king
The integration of AI and ML technologies with real-time transactional data is not merely an enhancement of existing financial services but a structural shift towards more secure, personalized, efficient and possibly even visionary banking. As these technologies continue to evolve, their potential to transform the financial sector grows exponentially. By embracing AI and ML, financial institutions can navigate the complexities of the digital age, delivering innovative services that meet the ever-changing needs of their customers. But we must always remember that without full data availability, these technologies will be starved of the information they need to minimise risk, protect against fraud and drive innovation. So many core banking technologies cannot provide the data needed to allow financial institutions to leverage AI, so for those wishing to stay at the forefront of innovation, a review of their current core is essential or there is a very real risk of being left behind.