AI is supercharging insight – but can your data keep up?
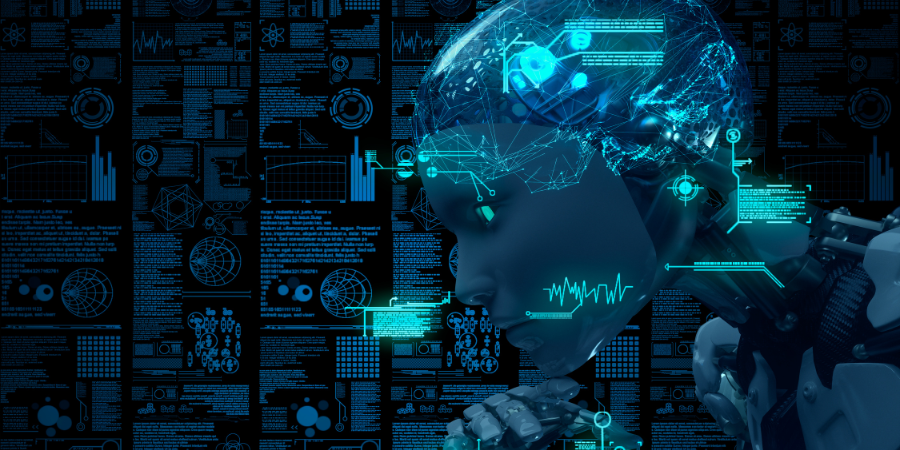
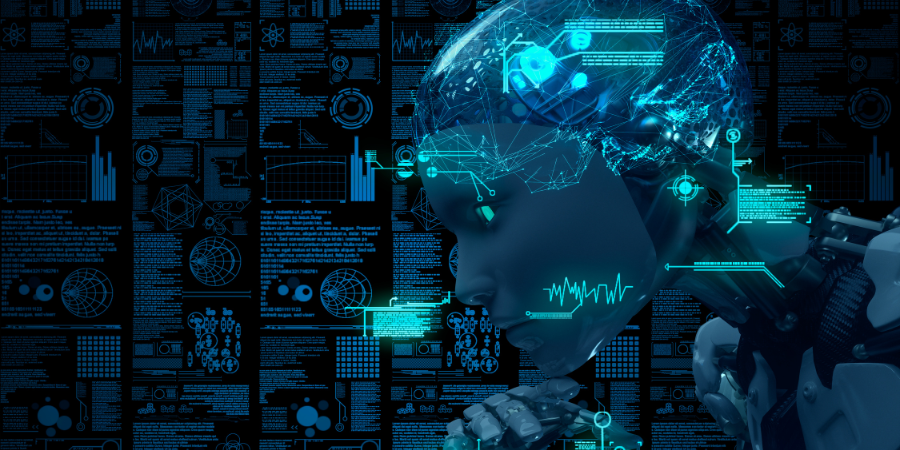
AI is being discussed by everyone in the tech world right now. With everything from coding, to copywriting, to photography and data visualisations, AI can often process in mere seconds things that once took hours, days or even weeks to do.
A recent example being discussed on social media was a CSV file listing lighthouse locations, being loaded into Code Interpreter in ChatGPT with an instruction to create a GIF showing the lighthouses twinkling against a dark backdrop. Within seconds an animated GIF was generated showing the coastline of the USA alive with the twinkling lights of hundreds of lighthouses. Just think about that for a moment. Code Interpreter was able to take basic data from a CSV file and overlay this on a map as specified by the user to create an animation – in seconds!
AI relies on good data
The big issue with AI is that it is only as good at the data available to it. For all of us who have tried AI technologies, we’ve had some ‘interesting’ outcomes where things have gone awry because of suspect or out-of-date source data. So having access to accurate real-time data is now more important than ever. With real-time data, businesses now have the ability to gather insights like never before. Perhaps a challenger fintech is workshopping new ideas for targeting their key markets – by accessing data on card use of their early adopters they can use AI to identify trends almost instantly and make decisions about marketing promotions at similar events or locations without having to wait weeks for data analysis. So if their cards have a big following with the festival crowd for example – there is no need to wait ‘til the next festival season to target like-minded festival folk.
Data architecture
This understanding of the importance of processing data at scale is the very reason that data architecture is a key consideration when choosing a core banking platform. AI tools are designed to understand and analyse complex data sets, extracting valuable insights and enabling real-time decision-making. A core banking solution must deliver a robust, scalable, and real-time data platform to leverage the benefits of AI, and it must do so in such a way that it allows tools such as Code Interpreter to access and analyse data in real-time without affecting the performance and throughput of new transactions. For instance, an AI tool could analyse real-time transaction data to detect fraudulent activity. By finding unusual patterns as they occur, the AI tool can alert the bank immediately, potentially stopping fraudulent transactions before they are completed.
By utilising architecture such as Command Query Responsibility Segregation and event-sourcing, the capabilities of AI tools can be greatly enhanced. By capturing all changes to the application state as a series of immutable events, complete audit history of data changes is available. This offers greater precision and traceability when analysing transactions and a rich data source that can be combined with existing machine learning models or used to train new ones.
Putting data to work
Consider a scenario where a bank wants to understand the spending habits of its customers. With our event sourcing architecture, an AI tool can analyse the complete history of a customer’s transactions, providing detailed insights into their spending patterns. These insights can then be used to offer personalised banking services, such as tailored loan offers or investment advice based on a recommendation model trained using the bank’s historic loan account transaction data. It can even be used to spot behaviours such as discontinued Direct Debits, reduced transactions or perhaps no longer receiving their pay through an account. This may be a red flag that the customer is planning to leave. Equally, changes in spending patterns or borrowing across a customer’s accounts can be an indicator that the customer is at risk of financial vulnerability which would allow the bank to act preemptively to offer assistance and prevent bad debt.
So by using an event-driven platform, AI tools can consume changes in application state in real-time. This means that business processes can be triggered at once with full operational context, rather than relying on inefficient nightly batch processing.
By making data architecture a key consideration in your choice of core banking solution you will have a robust platform for AI tools to understand and analyse data in real-time, not only improving the efficiency and performance of banking operations but also unlocking new possibilities for AI integration in the banking sector.
The landscape of banking is transforming. If you’re seeking to enhance your operations and adapt to these changes, genuine next-generation data-driven architecture must be considered essential for anyone planning to provide the financial products and services of the future.